About the project
Objective
Our planet faces unprecedented environmental challenges, including rapid urbanization, deforestation, pollution, loss of biodiversity, melting glacier, rising sea-level, and climate change. In recent years, the world also witnessed numerous natural disasters, from droughts, heat waves and wildfires to flooding and hurricanes, killing thousands and causing billions in property and infrastructural damages. With its synoptic view and large area coverage at regular revisits, satellite remote sensing has played a crucial role in monitoring our changing planet.
The overall objective of the EO-AI4GlobalChange (EO-AI4GlobalChange: Earth Observation Big Data and Deep Learning for Change Detection and Environmental Impact Assessment: Urbanization and Forest Fire Monitoring as Examples) project is to develop innovative and robust methods for monitoring global environmental changes using Earth Observation big data and deep learning. This research will focus on three major global environmental challenges: urbanization, wildfires and flooding.
Open and free Earth observation big data such as Sentionel-1 SAR and Sentinel-2 MSI data have been used to demonstrate the novel deep learning-based methods in selected cities worldwide and various wildfire and flooding sites across the globe. For urban mapping, a novel Domain Adaptation (DA) approach using semi-supervised learning has been developed for built-up area extraction. Several novel methods have been developed for urban change detection, including a dual-stream U-Net and a Siamese Difference Dual-Task network. For early detection of active fires, Gated Recurrent Units and transformer networks have been used to improve GOES-R and VIIRS dense time series detections.
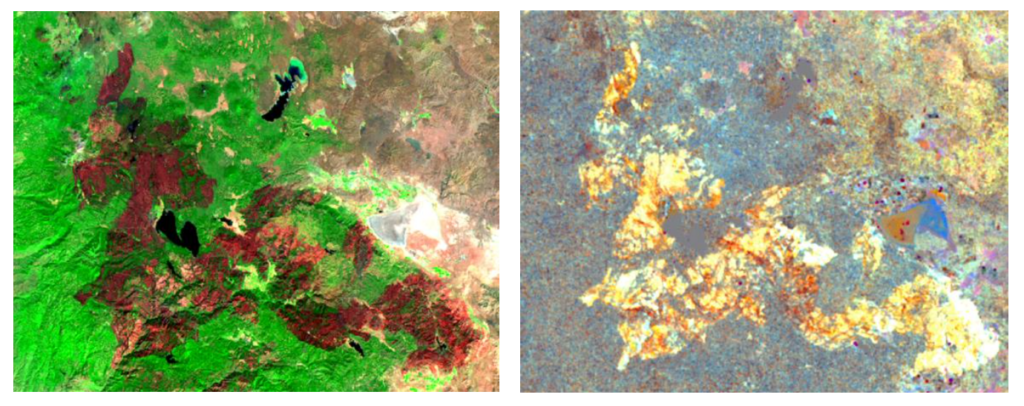
Figure 1. The Dixie Fire burned 963,309 acres (389,837 ha), was the largest single (i.e. non-complex) wildfire in recorded California history and the second-largest wildfire overall. The burned areas were clearly detected by the Sentinel-2 MSI (Left) and Sentinel-1 SAR (Right) images.
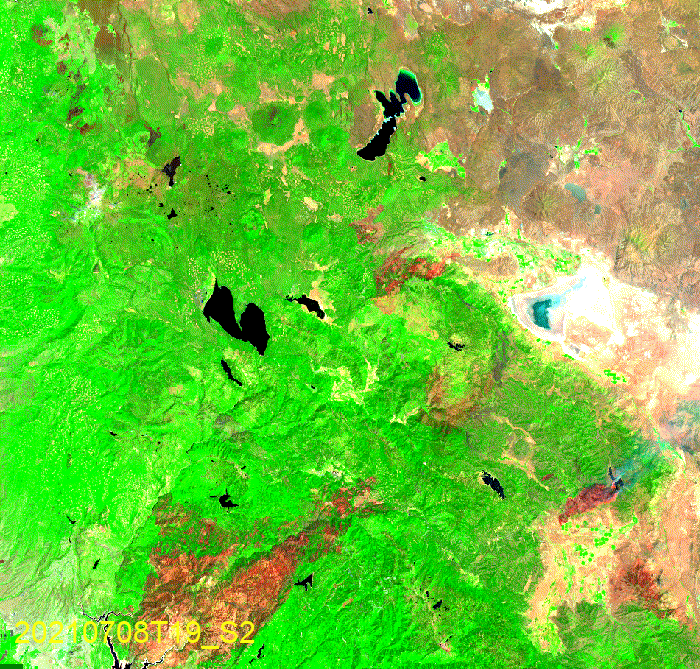
Figure 2. Started on July 13, 2021, and contained on October 25, 2021, the progression of the Dixie Fire was captured by Sentinel-1 and Sentinel-2 images.
For wildfire progression monitoring, transfer learning-based models have been evaluated to exploit Sentinel-1 SAR and Sentinel-2 MSI data. Civil contingencies agencies can use the timely and reliable information the project generates to support effective emergency management and decision-making during and after wildfires and flooding. Automatic and continuous mapping of urban areas and their changes can support sustainable and resilient city planning and contribute to monitoring the UN 2030 Urban Sustainable Development Goal (SDG 11).
Background
In recent years, the world has experienced many devastating wildfires due to human-induced climate change, most recently in Australia around the turn of 2019/2020. Wildfires kill and displace people, damage property and infrastructure, burn vegetation and harm wildlife, and cost billions of euros to fight. Up-to-date and reliable information on fire risk, active fires, fire extent, progression and damage assessment is critical for effective emergency management and decision support.
The pace of urbanization has been unprecedented. Rapid urbanization poses significant social and environmental challenges, including sprawling informal settlements, increased pollution, urban heat island, loss of biodiversity and ecosystem services, and making cities more vulnerable to disasters. Therefore, accurate and consistent information on urban changing patterns is essential to support sustainable urban development and the UN’s New Urban Agenda.
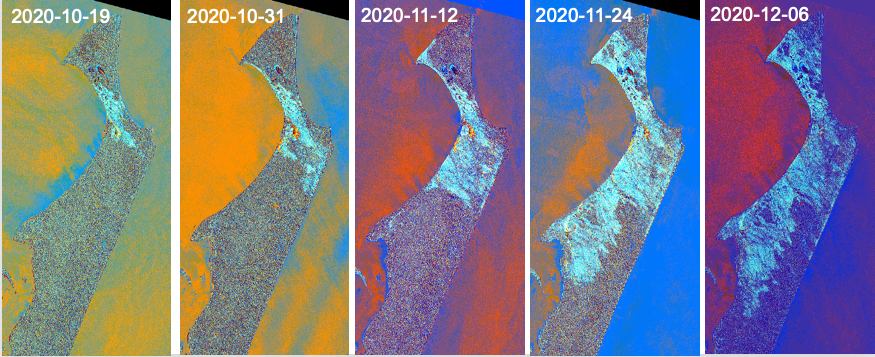
Activities & Results
Find out what’s going on!
Activities, awards, and other outputs
- Two Apps developed within this project, one for the SDG Indicator 11.3.1 Land Use Efficiency Calculation and the other for Urban Data Comparison, won the 2022 Group on Earth Observation (GEO)’s SDG Award at the GEO Plenary in early November in Ghana. (Note: GEO is a partnership of more than 100 national governments and in excess of 100 Participating Organizations that envisions a future where decisions and actions for the benefit of humankind are informed by coordinated, comprehensive and sustained Earth observations.)
- EO-AI4Wildfire within this project was selected among the Royal Swedish Engineering Academy’s 2020 Innovation 100 List.
Notable Presentations
- Ban, Y. 2022. invited speaker and panellist at “Implementing the Harmonized Global Urban Monitoring Framework (UMF)” and at “Training Sessions on EO4SDG Toolkit” at the 11th UN World Urban Forum, June 28-30, 2022, in Katowice, Poland, oral presentation, panel discussion, and training.
- Ban, Y. 2022. “EO-AI4GlobalChange” at the Geo for Good Lighting Talk Series #10 (virtual): Climate Action & Science, June 22, 2022, oral presentation.
- Ban, Y. 2022. “Earth Observation Big Data & AI for Monitoring Urban SDG Indicators”. ISPRS congress SDGs Forum, June 7, 2022, oral presentation and panel discussion (virtual).
- Mörtberg, U. 2022. Digital tools for ecological assessment of landcover changes. Digital Futures seminar: DF-Fly High Seminar, March 15th, 2022
- Ban, Y. 2021. EO-AI4GlobalChange, Keynote, the 28th International Conference on Geoinformatics, Nov. 2021, virtual.
- Ban, Y. 2021. EO4ResilientCities, UN COP26 Side Event “Earth Observations to build sustainable and climate resilient cities and communities”, Nov. 2021, virtual.
- Ban, Y. 2021. EO-AI4EnvironmentalChange, Invited speaker, Vinnova and Swedish National Space Agency’s event on Horizon Europe Cluster 4 – Digital, Industry and Space and Cluster 6 – Food, Bioeconomy, Natural Resources, Agriculture and Environment, May 26, 2021.
- Ban, Y. 2021. EO-AI4Wildfire at Google GEO for Good Virtual Summit 2021.
- Ban, Y. 2021. EO-AI4Wildfire at Google GEO for Good Seminar Series on Crisis Response.
- Ban, Y. 2020. EO-Enabled Global Urban Observation and Information to Support SDG and NUA, The 10th UN World Urban Forum, 2020, Abu Dhabi, UAE.
- Y. Ban. 2020-2021. EO-AI for Global Environmental Change Monitoring. Presented at KTH Space Rendezvous, Seminar Series of the International Space University, DF Fly-High, etc.
Conference Presentations
- Ban, Y., H. Azzizpour, A. Nascetti, J. Sullivan, U. Mörtberg. EO-AI4GlobalChange, ESA Living Planet Symposium, May 23-27, 2022, Bonn, Germany.
- Fang, H., Hao Hu, Andrea Nascetti, Yifang Ban, Hossein Azizpour. Multi-temporal Consistency Regularization for Change Detection on Satellite Imagery, ESA Living Planet Symposium, May 23-27, 2022, Bonn, Germany.
- Gerard, S. Y. Shi, D. Kerekes, Y. Ban, H. Azizpour, J. Sullivan. 2022. Critical Components of Strong Supervised Baselines for Building Damage Assessment in Satellite Imagery and their Limitations. ESA Living Planet Symposium, May 23-27, 2022, Bonn, Germany.
- Gerard, G. J. Sullivan. 2022. False temporal positives in self-supervised learning on satellite images. ESA Living Planet Symposium, May 23-27, 2022, Bonn, Germany.
- Hafner, S., Y. Ban and A. Nascetti, 2022. Urban Change Detection Using a Dual-Task Siamese Network and Semi-Supervised Learning. IGARSS’2022, Kuala Lumpur, Malaysia.
- Hu, X., P. Zhang and Y. Ban, 2022. Gan-Based SAR to Optical Image Translation in Fire-Disturbed Regions. IGARSS’2022, Kuala Lumpur, Malaysia, virtual.
- Kerekes, D., A. Nascetti, Y. Ban. 2022. Object detection methods for dark vessel detection and classification using SAR imagery. Living Planet Symposium, May 23-27, 2022, Bonn, Germany.
- Yadav, R., A. Nascetti and Y. Ban, 2022. Flood Detection and Mapping using Customized U- net Architectures based on Sentinel-1 SAR Imagery, Living Planet Symposium, May 23-27, 2022, Bonn, Germany.
- Yadav, R., A. Nascetti and Y. Ban, 2022. Building Change Detection Using Multi-Temporal Airborne Lidar Data. The 2022 ISPRS, June 6-11, 2022, Nice, France.
- Zhang, P., X. Hu, and Y. Ban, 2022. Wildfire-S1S2-Canada: A Large-Scale Sentinel-1/2 Wildfire Burned Area Mapping Dataset Based on the 2017-2019 Wildfires in Canada. IGARSS’2022, Kuala Lumpur, Malaysia, virtual.
- Zhao, Y. and Y. Ban, 2022. Global Scale Burned Area Mapping Using Bi-Temporal ALOS-2 PALSAR-2 L-Band Data. IGARSS’2022, Kuala Lumpur, Malaysia, virtual.
- Zhao, Y. and Y. Ban, 2022. VIIRS Time-series for wildfire progression mapping using Transformer Network. ESA Living Planet Symposium, May 23-27, 2022, Bonn, Germany.
- Yadav, R., A. Nascetti and Y. Ban, 2022. Attentive Dual Stream Siamese U-net for Flood Detection on Multi-temporal Sentinel-1 Data. IGARSS’2022, Kuala Lumpur, Malaysia, virtual.
- Hafner, S., Y. Ban and A. Nascetti, 2021. Exploring the Fusion of Sentinel-1 SAR and Sentinel-2 MSI Data for Built-Up Area Mapping Using Deep Learning. Proceedings of IGARSS’2021, Brussels, Belgium.
- Zhao, Y., Y. Ban and A. Nascetti, 2021. Early Detection of Wildfires with GOES-R Time-Series and Deep GRU Network. Proceedings of IGARSS’2021, Brussels, Belgium.
- Pang, X., Zhang, P., Mörtberg, U., Ban, Y. 2022. Ecological impacts of wildfire severity and pyrodiversity quantified with remote sensing and deep learning. Poster presentation at the 41th EARSeL Symposium, 13-16 September 2022, Paphos, Cyprus.
- Pang, X., Georganos, S., et al. 2022. Using remote sensing data with machine learning to predict distribution of near-threatened forest species. Poster presentation at the 41th EARSeL Symposium, 13-16 September 2022, Paphos, Cyprus.
- Pang, X.-L. 2021. Ecological impact of forest wild-fires vs clear-cuts—Digitalization on forest habitat networks and virtual species. Digital Futures seminar: DF-Dive Deep Seminar, October 14th, 2021, digital (https://www.youtube.com/watch?v=bTDEhlD8XEo).
- Pang, X.-L. 2022. Bridging the gap between essential biodiversity variables and indicators for forest species diversity detection by using remote sensing data. Oral presentation at Internationalization project of Visiting Tokyo University, 3rd Feb 2022, digital.
Results
The overall objective of the EO-AI4GlobalChange project is to develop innovative and robust methods for monitoring environmental changes using Earth Observation big data and deep learning. This research will focus on three major global environmental challenges: urbanization, wildfires and flooding.
The specific objectives are:
- to develop novel, automatic and globally applicable methods for effective change detection using deep learning and big data analytics to exploit all available Earth Observation data;
- to adapt the developed methods for continuous urban change detection, for flood mapping, and for wildfire monitoring including early detection of active fires, near real-time monitoring of wildfire progression and rapid damage estimation;
- to assess the environmental impacts of urbanization and wildfires on biodiversity and ecosystem services.
The research achievements are:
- Change Detection Methodology Development
- Change detection methods based on Segmentation networks with better generalization
- Temporal change detection using continuous observation
- Deep Learning for Environmental Change Monitoring
- Urban mapping and change detection
- Wildfire detection and monitoring
- Flood mapping
- Environmental impact assessment
List of open-data repositories and developed software
- We have started to collect historical wildfire data and satellite images to prepare a large-scale wildfire dataset.
- We have started collecting the reference and satellite images to prepare a large-scale 3D change detection dataset.
Publications
We like to inspire and share interesting knowledge!
Peer-reviewed journal publications and book chapter
- Hafner, S. Y. Ban and A. Nascetti, 2022. Unsupervised Domain Adaptation for Global Urban Extraction Using Sentinel-1 and Sentinel-2 Data. Remote Sensing of Environment. Vol. 280,113192, https://doi.org/10.1016/j.rse.2022.113192.
- Hafner, S., A. Nascetti, H. Azizpour and Y. Ban, 2022. Sentinel-1 and Sentinel- 2 Data Fusion for Urban Change Detection Using a Dual Stream U-Net. IEEE Geoscience and Remote Sensing Letters, Vol. 19, pp. 1-5. doi: 10.1109/LGRS.2021.3119856.
- Mugiraneza, T., S. Hafner, J. Haas, Y. Ban. 2022. Monitoring urbanization and environmental impact in Kigali, Rwanda using Sentinel-2 MSI data and ecosystem service bundles. International Journal of Applied Earth Observation and Geoinformation, Vol. 109, 102775. DOI: https://doi.org/10.1016/j.jag.2022.102775.
- Yadav, R., A. Nascetti, Y. Ban. 2022. Deep Attentive Fusion network for Flood Detection on Uni-temporal Sentinel-1 Data. Frontiers in Remote Sensing, section Microwave Remote Sensing (Accepted).
- Hu, X., Y. Ban, and A, Nascetti. 2021. Uni-Temporal Multispectral Imagery for Burned Area Mapping with Deep Learning. Remote Sensing, 13, no. 8: 1509.
- Hu, X., Y. Ban, and A, Nascetti. 2021. Sentinel-2 MSI data for active fire detection in major fire-prone biomes: A multi-criteria approach. International Journal of Applied Earth Observation and Geoinformation, 101, 102347.
- Zhang, P., Y. Ban, and A. Nascetti. 2021. Learning U-Net without Forgetting for Near Real-Time Wildfire Monitoring by the Fusion of SAR and Optical Time Series. Remote Sensing of Environment, 1-12. https://doi.org/10.1016/j.rse.2021.112467.
- Zhao Y, and Y. Ban. 2022. GOES-R Time Series for Early Detection of Wildfires with Deep GRU-Network. Remote Sensing. 2022; 14(17):4347. https://doi.org/10.3390/rs14174347.
- Furberg, D., and Y. Ban. 2021. Satellite monitoring of urbanization and environmental impacts in Stockholm, Sweden, through a multiscale approach. Urban Remote Sensing, 2nd Edition, Ed: X. Yang, Wiley.
- Furberg, D., Ban, Y. & Mörtberg, U., 2020. Monitoring urban green infrastructure changes and impact on habitat connectivity using high-resolution satellite data. Remote Sensing, 12(18), 3072. https://doi.org/10.3390/rs12183072.
- Ban, Y., Zhang, P., Nascetti, A., Bevington, A. R., Wulder, M. A., 2020. Near Real-Time Wildfire Progression Monitoring with Sentinel-1 SAR Time Series and Deep Learning. Scientific Reports, 10(1), 1–15. https://www.nature.com/articles/s41598-019-56967-x.
Peer-Reviewed Conference Papers
- Hafner, S., Y. Ban and A. Nascetti, 2022. Urban Change Detection Using a Dual-Task Siamese Network and Semi-Supervised Learning. Proceedings of IEEE International Geoscience and Remote Sensing Symposium (IGARSS) 2022, Kuala Lumpur, Malaysia.
- Hu, X., P. Zhang and Y. Ban, 2022. Gan-Based SAR to Optical Image Translation in Fire- Disturbed Regions. Proceedings of IGARSS’2022, Kuala Lumpur, Malaysia.
- Zhang, P., X. Hu, and Y. Ban, 2022. Wildfire-S1S2-Canada: A Large-Scale Sentinel-1/2 Wildfire Burned Area Mapping Dataset Based on the 2017-2019 Wildfires in Canada. Proceedings of IGARSS’2022, Kuala Lumpur, Malaysia.
- Zhao, Y., Y. Ban, 2022. Global Scale Burned Area Mapping Using Bi-Temporal ALOS-2 PALSAR-2 L-Band Data. Proceedings of IGARSS’2022, Kuala Lumpur, Malaysia.
- Yadav, R., A. Nascetti and Y. Ban, 2022. “Attentive Dual Stream Siamese U-net for Flood Detection on Multi-temporal Sentinel-1 Data,” Proceedings, IGARSS’2022.
- Yadav, R., Y. Ban and A. Nascetti, 2022. “Building Change Detection using Multi-Temporal Airborne LiDAR Data,” Proceedings, XXIV ISPRS Congress (2022 edition), 6–11 June 2022, Nice, France.
- Hafner, S., Y. Ban and A. Nascetti, 2021. Exploring the Fusion of Sentinel-1 SAR and Sentinel-2 MSI Data for Built-Up Area Mapping Using Deep Learning. Proceedings of IGARSS’2021, Brussels, Belgium.
- Zhao, Y., Y. Ban and A. Nascetti, 2021. “Early Detection of Wildfires with GOES-R Time- Series and Deep GRU Network,” Proceedings of IGARSS’2021, Brussels, Belgium.