About the project
Objective
The Explainable and Ethical Machine Learning for Knowledge Discovery from Medical Data Sources (EXTREMUM) project aims to develop a novel platform for learning from complex medical data sources with a focus on two healthcare application areas: adverse drug event detection and early detection and treatment of cardiovascular diseases.
The team will present a new framework for data management and analysis of data integration, methods for machine learning, and ethical issues related to predictive models. This project’s fundamental breakthrough is establishing a novel knowledge management and discovery framework for medical data sources. The outcome will be a set of methods and tools for integrating complex medical data sources, a set of predictive models for learning from these sources emphasising interpretability and explanatory features, and simultaneously focusing on maintaining ethical integrity in the underlying decision mechanisms that rule machine learning.
Background
One of the biggest challenges that research and business entities have been facing recently is that the data provided by today’s technologies originate from multiple data sources in massive quantities and at rapid rates. In addition to their volume, such data sources are complex and heterogeneous. In the presence of these complex and continuously growing information sources, domain scientists, data analysts, and novice users have been struggling to manage this complexity and arrive from abundant data to usable and interpretable models and exploitable domain knowledge. Towards this end, these data sources need to be monitored in real-time. Hence, data integration indexing and predictive modelling have become a major challenge. Consider, for example, the healthcare domain, where numerous data sources in the form of Electronic Health Records (EHRs), such as billing codes, registry data, and pharmaceutical data, are used for developing predictive models of, e.g., heart failure prevention and treatment progression, or adverse drug effect (ADE) detection.
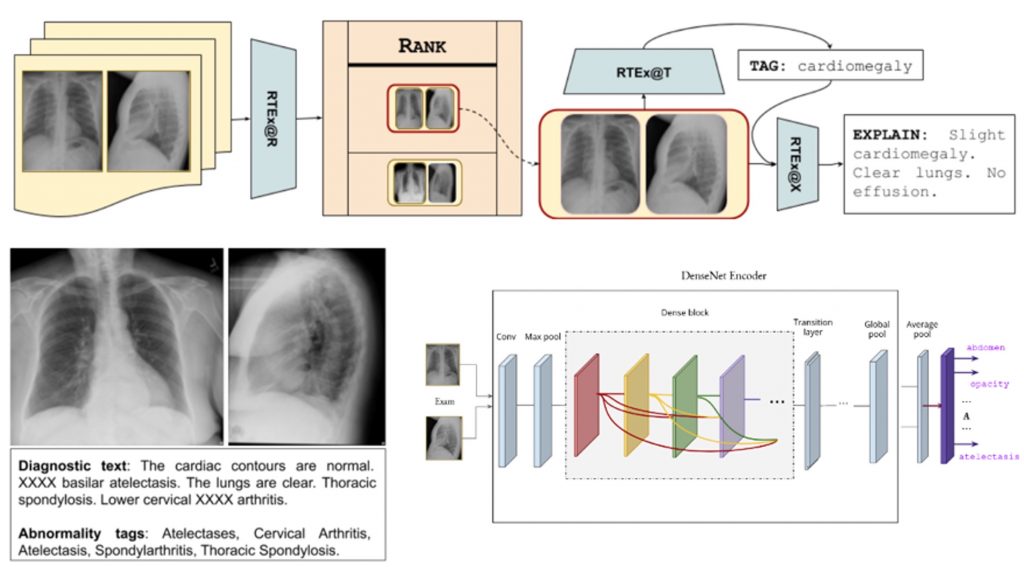
An example workflow of the EXTREMUM framework starts from radiography exams, ranking them based on their severity and urgency, then moving on to their classification and tagging while eventually producing clinically relevant explanatory text.
Cross-disciplinary collaboration
The project is a collaborative effort between four research institutions: the Department of Computer and Systems Sciences at Stockholm University, the Department of Law at Stockholm University, RISE Research Institutes Sweden, Division ICT, and the Department of Automatic Control, School of Electrical Engineering and Computer Science (EECS, KTH).
Watch the recorded presentation at the Digitalize in Stockholm 2023 event:
Activities & Results
Find out what’s going on!
Activities, awards, and other outputs
- XXXVI Nordic Conference on Law and Information Technology, ‘Securitization, Risk, Rule of Law – and, oh yes, a Pandemic!’, 8-10 November, 2021, Oslo. Presentation of the EXTREMUM project.
- Quality of Reasoning in Automated Judicial Decisions Conference, Örebro University, 14-15 February, 2022, Örebro. Presentation of the EXTREMUM project in relation to the conference theme.
- Business in Democracy Initiative (BiDEM) day-long conference, Copenhagen Business School, 6 April, 2022, Copenhagen. Presentation of the EXTREMUM project in relation to the conference theme.
- ECML/PKDD 2022: Presentation of one main conference paper (C1) and our demo paper (D1)
- MedAI PhD Forum 2022 (two-day workshop, Sep 6-7): Presentation of EXTREMUM to the Forum partners and discussion of potential synergies with Brunel University, University of Porto, and University of Magdeburg
- Joint Workshop between Stockholm University, KTH, and University of Manchester on explainable machine learning for healthcare (Sep 27-28 2021)
Results
The main goal of this project is to develop and establish a novel data representation, integration, and knowledge discovery framework for medical data sources, focusing on explainable machine learning governed by legal and ethical principles. Particular emphasis will be given to healthcare data sources available in EHRs, emphasising two particular healthcare problems: (a) early prediction and treatment of cardiovascular diseases and (b) adverse drug event identification and prevention.
Project objectives, along with the current achievements and results:
Objective 1: Unified representation and integration of complex data spaces. We have explored and defined novel space representations, similarity measures, and methods for searching and indexing large and complex data spaces for complex data sources. The basic challenge is the temporal nature of the data spaces and the inherent temporal dependencies that may exist within the same and across different data sources in these spaces. Our main solutions include the adoption and employment of temporal abstractions for temporal event sequences (both time series and discrete event sequences) of univariate and multivariate events.
Objective 2: Explainable predictive models for complex data sources. Regarding the second objective, our intention is to develop novel predictive modelling algorithms that can support and exploit data sources of complex nature and heterogeneity while at the same time providing explainability of their predictions in the form of interpretable features or rule sets. Towards this end, we have developed new methods for explainable machine learning with an emphasis on example-based explanations, such as counterfactuals for time series counterfactuals, as well as for event sequences). Moreover, we have explored model-based explanations and more concretely local explanations (such as LIME and SHAP), their applicability to the medical domain, and to what extent they are trusted and can be adopted by medical practitioners. In the case of time series data, interpretability can be achieved by focusing on white-box models, such as those described by differential equations, for which we have developed and analyzed several estimation algorithms.
We have also developed novel methods to reverse engineer predictors/filters for Hidden Markov Models and Linear Dynamical Systems, rendering them explainable by “opening the box”; furthermore, by modifying the samples arriving at a learning algorithm, we are developing methods that can improve learning while preserving privacy, enforcing fairness and arriving at more interpretable models. Our future plan is to develop similar methods for counterfactual generation for forecasting (e.g., for predicting critical events in the ICU and preventing them by suggesting critical actions. Furthermore, we intend to conduct a more extensive qualitative study on the generated explanations and counterfactuals involving a larger group of medical practitioners. This will also involve a qualitative assessment and deployment of our current demonstrator tool (published recently in the hospital setting on actual use cases.
Objective 3: Adherence to legal and ethical frameworks. We have focused on the legal and ethical implications associated with the development and use of predictive modelling in relation to healthcare data analysis. To this end, the General Data Protection Regulation (GDPR) is of utmost relevance. We have identified the predominant social values promoted in the GDPR and transposed these legal rules into mathematical equivalents. The finding from this process has been interesting to the extent that the identified social values are mutually exclusive and that promoting one of them occurs at the expense of the others, necessitating a balancing act. The social values studied thus far were privacy, accuracy and explainability. The results of this examination are relevant from a legislative techniques perspective, culminating in these interdisciplinary findings being published in a legal publication venue.
Publications
We like to inspire and share interesting knowledge!
Journal articles (J)
- R.A.González,C.R.Rojas,S.Pan,andJ.Welsh.“Theoreticalandpracticalaspects of the convergence of the SRIVC estimator for over-parameterized models”. Automatica, 142:110355, 2022
- S. Pan, J. Welsh, R. A. González, and C. R. Rojas. “Consistency analysis and bias elimination of the instrumental variable based state variable filter method”. Automatica, 144:110511, 2022
- P. Wachel and C. R. Rojas. “An adversarial approach to adaptive model predictive control”. Journal of Advances in Applied & Computational Mathematics, 9, 135–146, 2022
- R. A. González, C. R. Rojas, S. Pan, and J. Welsh. “Refined instrumental variable methods for unstable continuous-time systems in closed-loop”. International Journal of Control (accepted for publication), 2022
- C.R.RojasandP.Wachel.“Onstate-spacerepresentationsofgeneraldiscrete-time dynamical systems”. IEEE Transactions on Automatic Control (accepted for publication), 2022
- R.Mochaourab,A.Venkitaraman,I.Samsten,P.Papapetrou,andC.R.Rojas,“Post- hoc Explainability for Time Series Classification: Toward a Signal Processing Perspective,” IEEE Signal Processing Magazine, Special Issue on Explainability in Data Science: Interpretability, Reproducibility, and Replicability, vol. 39, no. 4, Jul. 2022
- S. Greenstein, P. Papapetrou, and R. Mochaourab, “Embedding Human Values into Artificial Intelligence,” in De Vries, Katja (ed.), De Lege, Iustus förlag, Uppsala University, 2022
- J. Rebane, I. Samsten, L. Bornemann, and P. Papapetrou, “SMILE: A feature-based temporal abstraction framework for event-interval sequence classification”. In Data Mining and Knowledge Discovery (DAMI) 35(1): 372-399, 2021
- B. Djehiche, O. Mazhar, and C. R. Rojas. “Finite impulse response models: A nonasymptotic analysis of the least squares estimator”. Bernoulli, 27(2):976–1000, 2021
- R. A. González, C. R. Rojas, S. Pan, and J. Welsh. “Consistent identification of continuous-time systems under multisine input signal excitation”. Automatica, 133:109859, 2021
- I. Lourenço, R. Mattila, C. R. Rojas, X. Hu, and B.Wahlberg. “Hidden Markov models: Inverse filtering, belief estimation and privacy protection”. Journal of Systems Science and Complexity, 34:1801–1820, 2021
- J. Rebane, I. Samsten, and P. Papapetrou, “Exploiting Complex Medical Data with Interpretable Deep Learning for Adverse Drug Event Prediction”. In Artificial Intelligence in Medicine (AIM), 28(8): 1651-1659, 2021
- Locally and globally explainable time series tweaking KAIS), 62 (5): 1671- 1700, 2020
- R. Mattila, C. R. Rojas, V. Krishnamurthy, and B. Wahlberg. “Inverse filtering for hidden Markov models with applications to counter-adversarial autonomous systems”. IEEE Transactions on Signal Processing, 68:4987–5002, 2020
Conference papers (C)
- Z. Lee, M. Trincavelli, and P. Papapetrou, “Finding Local Groupings of Time Series“, In the European Conference on Machine Learning and Principles and Practices of Knowledge Discovery in Databases (ECML/PKDD), to appear
- L. Mondrejevski, I. Miliou, A. Montanino, D. Pitts, J. Hollmén, and P. Papapetrou, “FLICU: A Federated Learning Workflow for Intensive Care Unit Mortality Prediction”. In Computer-Based Medical Systems (CBMS), to appear
- M. Bampa, T. Fasth, S. Magnusson, and P. Papapetrou, “EpidRLearn: Learning intervention strategies for epidemics with Reinforcement Learning“. In the International Conference on Artificial Intelligence in Medicine (AIME), 2022
- S. Guarnizo, I. Miliou, and P. Papapetrou, “Impact of Dimensionality on Nowcasting Seasonal Influenza with Environmental Factors“. In the International Symposium on Intelligent Data Analysis (IDA), 128-142, 2022
- J. Parsa, C. R. Rojas, and H. Hjalmarsson. “Optimal input design for sparse system identification”. In Proceedings of the 2022 European Control Conference (ECC), 2022
- B. Lakshminarayanan and C. R. Rojas. “A statistical decision-theoretical perspective on the two-stage approach to parameter estimation”. In Proceedings of the 61th IEEE Conference on Decision and Control (CDC 2022) (accepted for publication), 2022
- I. Lourenço, R. Winqvist, C. R. Rojas, and B. Wahlberg. “A teacher-student Markov decision process-based framework for online correctional learning”. In Proceedings of the 61th IEEE Conference on Decision and Control (CDC 2022) (accepted for publication), 2022
- Z. Wang, I. Samsten, and P. Papapetrou, Counterfactual Explanations for Survival Prediction of Cardiovascular ICU Patients. In Artificial Intelligence in Medicine (AIME), 338-348, 2021 [best student paper award]
- J.PavlopoulosandP.Papapetrou,CustomizedNeuralPredictiveMedicalText:AUse- Case on Caregivers. In Artificial Intelligence in Medicine (AIME), 438-443, 2021
- J. Rebane, I. Samsten, P. Pantelidis, and P. Papapetrou, Assessing the Clinical Validity of Attention-based and SHAP Temporal Explanations for Adverse Drug Event Predictions. In Computer-based Medical Systems (CBMS), 235-240, 2021
- Z. Wang, I. Samsten, R. Mochaourab, and P. Papapetrou, Learning Time Series Counterfactuals via Latent Space Representations. In Discovery Science (DS), 369- 384, 2021
- I. Lourenço, R. Mattila, C. R. Rojas, and B. Wahlberg. “Cooperative system identification via correctional learning”. In Proceedings of the 19th IFAC Symposium on System Identification (SYSID 2021), 2021
- R. A. González, C. R. Rojas, and H. Hjalmarsson. “Non-causal regularized least- squares for continuous-time system identification with band-limited input excitations”. In Proceedings of the 60th IEEE Conference on Decision and Control (CDC 2021), 2021
- R. A. González, C. R. Rojas, S. Pan, and J. S. Welsh. “The SRIVC algorithm for continuous-time system identification with arbitrary input excitation in open and closed loop”. In Proceedings of the 60th IEEE Conference on Decision and Control (CDC 2021), 2021
- Z. Lee, T. Lindgren, and P. Papapetrou, “Z-Miner: an efficient method for mining frequent arrangements of event intervals“. In ACM Knowledge Discovery and Data Mining (KDD), 524-534, 2020
- Z. Lee, S. Girdzijauskas, and P. Papapetrou, “Z-Embedding: A spectral representation of event intervals for efficient clustering and classification“. In the European Conference on Machine Learning and Principles and Practices of Knowledge Discovery in Databases (ECML/PKDD), 710-726, 2020
- M. Bampa, P. Papapetrou, and J. Hollmen, “A clustering framework for patient phenotyping with application to adverse drug events“. In IEEE Computer-Based Medical Systems (CBMS), 177-182, 2020
- J. Pavlopoulos and P. Papapetrou, “Clinical predictive keyboard using statistical and neural language modeling“. In IEEE Computer-Based Medical Systems (CBMS), 293- 296, 2020
- Z. Lee, J. Rebane, and P. Papapetrou, “Mining disproportional frequent arrangements of event intervals for investigating adverse drug events“. In IEEE Computer-Based Medical Systems (CBMS), 293-296, 2020
- N. B. Kumarakulasinghe, T. Blomberg, J. Liu, A. S. Leao and P. Papapetrou, “Evaluating local interpretable model-agnostic explanations on clinical machine learning classification models“. In IEEE Computer-Based Medical Systems (CBMS), 7-12, 2020
- R. Mattila, I. Lourenço, V. Krishnamurthy, C. R. Rojas, and B. Wahlberg. “What did your adversary believe? Optimal smoothing in counter-autonomous systems”. In Proceedings of the 45th International Conference on Acoustics, Speech, and Signal Processing (ICASSP 2020), Barcelona, Spain, 2020
- R. A. González and C. R. Rojas. “Finite sample deviation and variance bounds for first order autoregressive processes”. In Proceedings of the 45th International Conference on Acoustics, Speech, and Signal Processing (ICASSP 2020), Barcelona, Spain, 2020
- R. A. González and C. R. Rojas. “A finite-sample deviation bound for stable autoregressive processes”. In Proceedings of the 2nd Conference on Learning for Decision and Control (L4DC), Berkeley, USA, 2020
- R. A. González, J. S. Welsh, and C. R. Rojas. “Enforcing stability through ellipsoidal inner approximations in the indirect approach for continuous-time system identification”. In Proceedings of the 21st IFAC World Congress (IFAC 2020), Berlin, Germany, 2020
- R. Mattila, C. R. Rojas, E. Moulines, V. Krishnamurthy, and B.Wahlberg. “Fast and consistent learning of hidden Markov models by incorporating non-consecutive correlations”. In Proceedings of the 37th International Conference on Machine Learning (ICML 2020), 2020
- I. Lourenço, R. Mattila, C. R. Rojas, and B. Wahlberg. “How to protect your privacy? A framework for counter-adversarial decision making”. In Proceedings of the 59th IEEE Conference on Decision and Control (CDC 2020), 2020
- R. Mochaourab, S. Sinha, S. Greenstein, and P. Papapetrou, “Robust Counterfactual Explanations for Privacy-Preserving SVMs,” International Conference on Machine Learning (ICML 2021), Workshop on Socially Responsible Machine Learning, Jul. 2021
Demo paper (D)
- R. Mochaourab, S. Sinha, S. Greenstein, and P. Papapetrou, “Demonstrator on Counterfactual Explanations for Differentially Private Support Vector Machines,” European Conference on Machine Learning and Principles and Practice of Knowledge Discovery in Databases (ECML PKDD 2022), Demo Track, Sept. 2022