About the project
Objective
In the DataLEASH project, practically, we develop and test machine learning models, among other methods, to ensure the use of data without the risk of revealing people’s identities or allowing unwanted inferences about them. In a more theoretical approach, we aim at provable guarantees for privacy and take a holistic approach to the legal implications. This implies a quest for finding relevant rules and regulations and illuminating interpretation and application.
The project consortium from KTH, SU, and RISE has a unique set-up in terms of an interdisciplinary and multidisciplinary profile among the researchers, combining perspectives from information theory, legal informatics, language processing, machine learning, cryptography, and systems security.
Background
Digitalization has resulted in more and more data being generated and collected from various sources (such as health care, customer service, surveillance cameras, etc.). The data is valuable for processing and additional analysis to improve predictions and planning. Advances in machine learning have improved this kind of data analysis, while data-protection regulation such as the GDPR has introduced constraints, limiting what data can be used and for what purpose. There is, thus a tension between the utility of data and the privacy of the individuals the data is about.
Cross-disciplinary collaboration
DataLEASH brings together researchers from the School of Electrical Engineering and Computer Science (EECS, KTH), the Department of Computer and Systems Sciences (DSV) and the Department of Law both at Stockholm University and from the Decisions, Network, and Analytics lab at RISE.
Watch the recorded presentation at Digitalize in Stockholm 2022 event:
Activities & Results
Activities, awards, and other outputs
- Speakers at workshops on “AI inom medicinteknik,” session “Vad minns en högparametriserad modell? Organized by Läkemedelsverket, April 6, online with more than 150 participants from industry and regulatory bodies.
- “Tillgängliggörande av hälsodata,” Dec 2021 online with more than 50 participants from four regions participating
- “Digital innovation i samverkan stad, region och akademi,” Oct 2021 online with about 20 participants from KTH, Region and City of Stockholm, plus some KTH internal events.
- Organisation and participation of panel at Nordic Privacy Forum 2022 panel discussing calculated privacy and the interplay between law and tech.
- DataLEASH organizes regular seminars every two months for three years with the City of Stockholm and Region Stockholm about requirements from the stakeholders and the results from our research project.
- SAIS 2022, Swedish AI Society workshop, is organised and paper [BFLSSR22] is presented in this workshop.
- Award: Rise solution for Encrypted Health AI was announced the winner of the Vinnova Vinter competition in the infrastructure category.
Results
Research objectives of DataLEASH are: (i) develop and study privacy measures suitable for privacy risk assessment and utility optimization; (ii) characterization of fundamental bounds on data disclosure mechanisms; (iii) design and study of efficient data disclosure mechanisms with privacy guarantees; (iv) demonstration and testing of algorithms using real-data repositories; (v) study of the cross-disciplinary privacy aspects between law and information technology.
Research achievements and main results of DataLEASH:
- Pointwise Maximal Leakage (PML) has been proposed as a new privacy measure framework. PML has an operational meaning and is robust. Using the framework, several other privacy measures have been derived and their properties have been characterized as well as the relation to existing privacy measures have been established.
- The privacy-preserving learning mechanism PATE has been studied using conditional maximal leakage explaining the cost of privacy. PATE approach has been extended to deal with high-dimensional targets such as in segmentation tasks of MRI brain scans.
- Fundamental bounds on data disclosure mechanisms have been derived considering various pointwise privacy measures. Furthermore, approximate solutions to optimal data disclosure mechanisms have been derived using concepts from Euclidian Information Theory.
- In a cross-disciplinary study between law and tech, we propose and discuss how to relate the legal data protection principles of data minimization to the mathematical concept of a sufficient statistic to be able to deal from a regulatory perspective with the rapid advancements in machine learning.
- Health Bank, a large health data repository of 2 million patient record texts in Swedish has been de-identified. A deep learning BERT model, SweDeClin-BERT, has been created and obtained permission from the Swedish Ethical Review Authority to be shared among academic users. The model SweDeClin-BERT has been used at the University Hospital of Linköping with promising results. Handling sensitive health-related data is often challenging. Proposed Fully Homomorphic Encryption (FHE) to encrypt diabetes data. The proposed approach won the pilot Winter competition 2021–22 organized by Vinnova.
- We created a systematization of knowledge on ambient assisted living (combining the challenges of mobile and smart-home monitoring for health) from a privacy perspective to map out potential issues and intervention points.
- Using a cryptographic approach, we developed distance-bounding attribute-based credentials, which provide anonymity for location-based services, provably resisting attacks.
- We investigated the uses and limitations of synthetic data as a privacy-preservation mechanism. For image data, we developed a framework of clustering and synthesizing facial images for privacy-preserving data analysis with privacy guarantees from k-anonymity and found trade-off choice points with analysis utility. In a different work on facial images, we proposed a novel approach for the privacy preservation of attributes using adversarial representation learning. This work removes the sensitive facial expressions and replaces them with an independent random expression while preserving facial features. For tabular data, we investigated across several datasets whether different methods of generating fully synthetic data vary in their utility a priori (when the specific analyses to be performed on the data are not known yet), how closely their results conform to analyses on original data a posteriori, and whether these two effects are correlated. We found classification tasks when using synthetic data for training machine-learning models more promising in terms of consistent accuracy than statistical analysis.
In the interplay between information technology and law, the project itself has been a testbed, given the personal data processing in research of this kind. Quite often, there is a challenge merely to find the governing legal framework. Practical experiences and theoretical studies can be a sign of this. However, much research today is concentrated on specific data protection regulations. The reasoning above boils down to a broadened approach to GDPR.
Publications
We like to inspire and share interesting knowledge…
- Vakili, T., Hullmann T., Henriksson A. and H. Dalianis. 2024. When Is a Name Sensitive? Eponyms in Clinical Text and Implications for De-Identification. To be presented at the CALD-pseudo Workshop at the 18th Conference of the European Chapter of the Association for Computational Linguistics, EACL 2024, Malta.
- Ngo, P., Tejedor M., Olsen Svenning T., Chomutare T., Budrionis A. and H. Dalianis. 2024. Deidentifying a Norwegian clinical corpus – An effort to create a privacy-preserving Norwegian large clinical language model. To be presented at the CALD-pseudo Workshop at the 18th Conference of the European Chapter of the Association for Computational Linguistics, EACL 2024, Malta.
- Lamproudis, A., Mora, S., Olsen Svenning T., Torsvik T., Chomutare T., Dinh Ngo P. and H. Dalianis. 2023. De-identifying Norwegian Clinical Text using Resources from Swedish and Danish. Proceedings of AMIA 2023, Annual Symposium, November 11-15. New Orleans, LA, USA, link.
- Vakili, T. and H. Dalianis. 2023. Using Membership Inference Attacks to Evaluate Privacy-Preserving Language Modeling Fails for Pseudonymizing Data. Proceedings of the 24th Nordic Conference on Computational Linguistics (NoDaLiDa 2023). Faroe Islands, May 22-24, 2023, link.
- Vakili, T., Lamproudis, A., Henriksson, A. and H. Dalianis. 2022. Downstream Task Performance of BERT Models Pre-Trained Using Automatically De-Identified Clinical Data. In the Proceedings of the 13th International Conference on Language Resources and Evaluation, LREC 2022, Marseille, France, pp. 4245–4252, link.
- Vakili, T. and H. Dalianis 2022, Utility Preservation of Clinical Text After De-Identification. In the Proceedings of the 21st Workshop on Biomedical Language Processing (pp. 383-388) in conjunction with ACL 2022, Dublin, Ireland, link.
- Sara Saeidian, Giulia Cervia, Tobias J. Oechtering, Mikael Skoglund, Quantifying Membership Privacy via Information Leakage, IEEE Transactions Information Forensics and Security. Vol.16, pp. 3096-3108, 2021, link.
- Sara Saeidian, Giulia Cervia, Tobias J. Oechtering, Mikael Skoglund, Optimal Maximal Leakage-Distortion Tradeoff. Information Theory Workshop (ITW) 2021 IEEE, pp. 1-6, 2021, link.
- Vakili, T. and H. Dalianis. 2021. Are Clinical BERT Models Privacy-Preserving? The Difficulty of Extracting Patient-Condition Associations. In the Proceedings of the Association for the Advancement of Artificial Intelligence AAAI Fall 2021 Symposium in HUman partnership with Medical Artificial iNtelligence (HUMAN.AI), November 4-6, 2021, pdf.
- Lamproudis, A., Henriksson, A. and H. Dalianis. 2021. Developing a Clinical Language Model for Swedish: Continued Pretraining of Generic BERT with In-Domain Data. In the Proceeding of RANLP 21: Recent Advances in Natural Language Processing, 1-3 Sept 2021, Varna, Bulgaria, pdf.
- Grancharova, M. and H. Dalianis. 2021. Applying and Sharing pre-trained BERT-models for Named Entity Recognition and Classification in Swedish Electronic Patient Records. In the Proceedings of the 23rd Nordic Conference on Computational Linguistics, NoDaLiDa 2021, Iceland, May 31 – June 2, 2021, pdf.
- Dalianis, H. and H. Berg. 2021. HB Deid – HB De-identification tool demonstrator. In the Proceedings of the 23rd Nordic Conference on Computational Linguistics, NoDaLiDa 2021, Iceland, May 31 – June 2, 2021, pdf.
- Berg, H., Henriksson, A., Fors, U. and H. Dalianis. 2021. De-identification of Clinical Text for Secondary Use: Research Issues. In the proceedings of HEALTHINF 2021, 14th International Conference on Health Informatics Feb 11-13, 2021, pdf.
- Grancharova, M., Berg, H. and H. Dalianis. 2020. Improving Named Entity Recognition and Classification in Class Imbalanced Swedish Electronic Patient Records through Resampling. Compilation of abstracts in The Eight Swedish Language Technology Conference (SLTC-2020), Göteborg, pdf.
- Berg, H., A.Henriksson and H. Dalianis. 2020. The Impact of De-identification on Downstream Named Entity Recognition in Clinical Text. In Proceedings of the 11th International Workshop on Health Text Mining and Information Analysis, Louhi 2020, in conjunction with EMNLP 2020, (pp. 1-11), pdf.
- Berg, H., Henriksson, A., Fors, U. and H. Dalianis. De-identification of Clinical Text for Secondary Use: Research Issues. Presented at the Healthcare Text Analytics Conference HealTAC 2020, April 23, London.
- Berg, H. and H. Dalianis. 2020. A Semi-supervised Approach for De-identification of Swedish Clinical Text. Proceedings of 12th Conference on Language Resources and Evaluation, LREC 2020, May 13-15, Marseille, pp. 4444‑4450, pdf.
- Berg, H., T. Chomutare and H. Dalianis. 2019. Building a De-identification System for Real Swedish Clinical Text Using Pseudonymised Clinical Text. In the Proceedings of the Tenth International Workshop on Health Text Mining and Information Analysis, Louhi 2019, in conjunction with Conference on Empirical Methods in Natural Language Processing, (EMNLP) November 2019, Hongkong, ACL, pp 118-125, pdf.
- Berg, H. and H. Dalianis. 2019. Augmenting a De-identification System for Swedish Clinical Text Using Open Resources (and Deep learning). In the Proceedings of the Workshop on NLP and Pseudonymisation, in conjunction with the 22nd Nordic Conference on Computational Linguistics (NoDaLiDa), Turku, Finland, September 30, 2019, pdf.
- Dalianis, H. 2019. Pseudonymisation of Swedish Electronic Patient Records Using a Rule-based Approach. In the Proceedings of the Workshop on NLP and Pseudonymisation, in conjunction with the 22nd Nordic Conference on Computational Linguistics (NoDaLiDa), Turku, Finland, September 30, 2019, pdf.
Videos & Presentations
Watch recorded videos and download the presentations…
VIDEO RECORDINGS
Presentation at Digitalize in Stockholm 2022
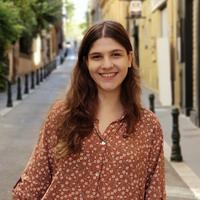
Research: Privacy-preserving data analysis. We apply tools from information theory to problems related to privacy-preserving data analysis
Speaker: Sara Saeidian, PhD student, saeidian@kth.se
Supervisors: Tobias J. Oechtering, Mikael Skoglund
Click here to watch the recorded video presentation on “Privacy-preserving data analysis”
OUR PRESENTATIONS
Quantifying Membership Privacy via Information Leakage
Sara Saeidian, Giulia Cervia, Tobias J. Oechtering, Mikael Skoglund, “Quantifying Membership Privacy via Information Leakage, IEEE Transactions Information Forensics and Security, Vol.16, pp. 3096-3108, 2021.
Machine learning models are known to memorize the unique properties of individual data points in a training set. This memorization capability can be exploited by several types of attacks to infer information about the training data, most notably, membership inference attacks. In this work, we propose an approach based on information leakage for guaranteeing membership privacy. Specifically, we propose to use a conditional form of the notion of maximal leakage to quantify the information leaking about individual data entries in a dataset, i.e., the entrywise information leakage.
We apply our privacy analysis to the Private Aggregation of Teacher Ensembles (PATE) framework for privacy-preserving classification of sensitive data and prove that the entrywise information leakage of its aggregation mechanism is Schur-concave when the injected noise has a log-concave probability density. The Schur-concavity of this leakage implies that increased consensus among teachers in labelling a query reduces its associated privacy cost. We also derive upper bounds on the entrywise information leakage when the aggregation mechanism uses Laplace distributed noise.
DOWNLOAD THE PRESENTATION HERE: Quantifying Membership Privacy via Information Leakage