Fusion of Radar and Optical Remote Sensing Time Series for Wildfire Monitoring with Deep Learning
November 2020 – October 2022
Objective
Wildfire monitoring involves two main problems, i.e., active fire detection and burnt area mapping. Active fire detection aims to find the ongoing wildfire hotspots, while burnt area mapping is expected to detect the areas affected by wildfire.
This project mainly focuses on large-scale wildfire burnt area mapping and near real-time wildfire monitoring. In view of the limited transfering performance of the existing wildfire monitoring algorithms on a larger scale and various climate zones, this project aims to develop large-scale or even globally applicable models by exploiting global coverage, multi-source satellite remote sensing data and advanced machine learning/deep learning techniques.
Background
Wildfire has coexisted with human societies for more than 350 million years, always playing an important role in shaping the Earth’s surface and climate. Across the globe, wildfires are becoming larger, more frequent, longer-duration, and tend to be more destructive in terms of lives lost and economic costs because of climate change and human activities.
To reduce the damages from such destructive wildfires, it is critical to track wildfire progressions in near real-time, or even in real-time, to support fire-fighting and keep everything under control. Satellite remote sensing enables cost-effective, accurate, and timely monitoring of the wildfire progressions over vast geographic areas. The free availability of global coverage Landsat-8, and Sentinel-1/2 satellite data opens a new era for global land surface monitoring, providing an opportunity to analyse wildfire impacts around the globe.
About the Digital Futures Postdoc Fellow
Puzhao Zhang is a research fellow at the Division of Geoinformatics, Royal Institute of Technology. He received his PhD in Pattern Recognition and Intelligent Systems from Xidian University, China, at the end of 2019. His PhD research was focused on remote sensing and deep learning for change detection.
Since fall of 2017, he has worked on wildfire monitoring with radar and optical remote sensing and deep learning as a joint PhD student at KTH. His research interests include satellite imagery analysis, change detection, machine learning, deep learning, and spatio-temporal modelling for monitoring environmental change and biomass carbon dynamics.
Main supervisor
Yifang Ban, Professor, Division of Geoinformatics, KTH
Co-supervisor
Josephine Sullivan, Associate professor, Division of Robotics, Perception and Learning, KTH
Watch the recorded presentation at Digitalize in Stockholm 2022 event:
Contacts
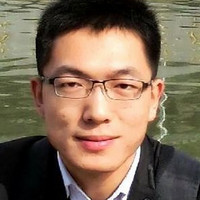
Puzhao Zhang
Former Digital Futures Postdoctoral Fellow, Postdoc project: Fusion of Radar and Optical Remote Sensing Time Series for Wildfire Monitoring with Deep Learning
puzhao@kth.se
Yifang Ban
Professor and Head of Division Geoinformatics at KTH, Member of the Executive Committee, Associate Director for Dissemination & Impact, PI: EO-AI4GlobalChange, Former Main supervisor: Unraveling the potential of AI and Earth Observation for accurate population predictions in urban regions (POPAI), Former Main supervisor: Fusion of Radar and Optical Remote Sensing Time Series for Wildfire Monitoring with Deep Learning, Digital Futures Faculty
+46 8 790 86 48yifang@kth.se

Josephine Sullivan
Associate Professor, Division of Robotics, Perception and Learning at KTH, Co-PI: EO-AI4GlobalChange, Former Co-Supervisor: Fusion of Radar and Optical Remote Sensing Time Series for Wildfire Monitoring with Deep Learning, Digital Futures Faculty
+46 8 790 61 36sullivan@kth.se